Common AI Implementation Challenges and How to Overcome Them
Introduction to AI Implementation
Artificial Intelligence (AI) is transforming industries by enhancing efficiency, improving decision-making, and offering personalized experiences. However, implementing AI comes with its own set of challenges that organizations must tackle to unlock its full potential. In this article, we explore some common AI implementation challenges and provide solutions to overcome them.

Data Management and Quality
One of the primary challenges in AI implementation is managing data quality and quantity. AI systems require vast amounts of data to function effectively, but if the data is inaccurate or incomplete, it can lead to unreliable outcomes. Ensuring data quality involves cleaning, labeling, and organizing data so it is suitable for analysis.
Solution: Establish Robust Data Practices
To overcome this challenge, organizations should establish robust data management practices. This includes implementing data validation processes, using data augmentation techniques, and continuously monitoring data integrity. Additionally, leveraging advanced data management tools can automate much of this process, ensuring high-quality input for AI systems.
Lack of Skilled Talent
The demand for skilled AI professionals often outpaces supply, making it difficult for organizations to find the right talent. Data scientists, machine learning engineers, and AI specialists are crucial for successful AI implementation but are often hard to come by.
Solution: Upskill and Collaborate
Organizations can address this talent gap by investing in upskilling their current workforce through training programs and workshops. Collaborating with academic institutions and engaging in partnerships with tech companies can also provide access to a broader pool of expertise.
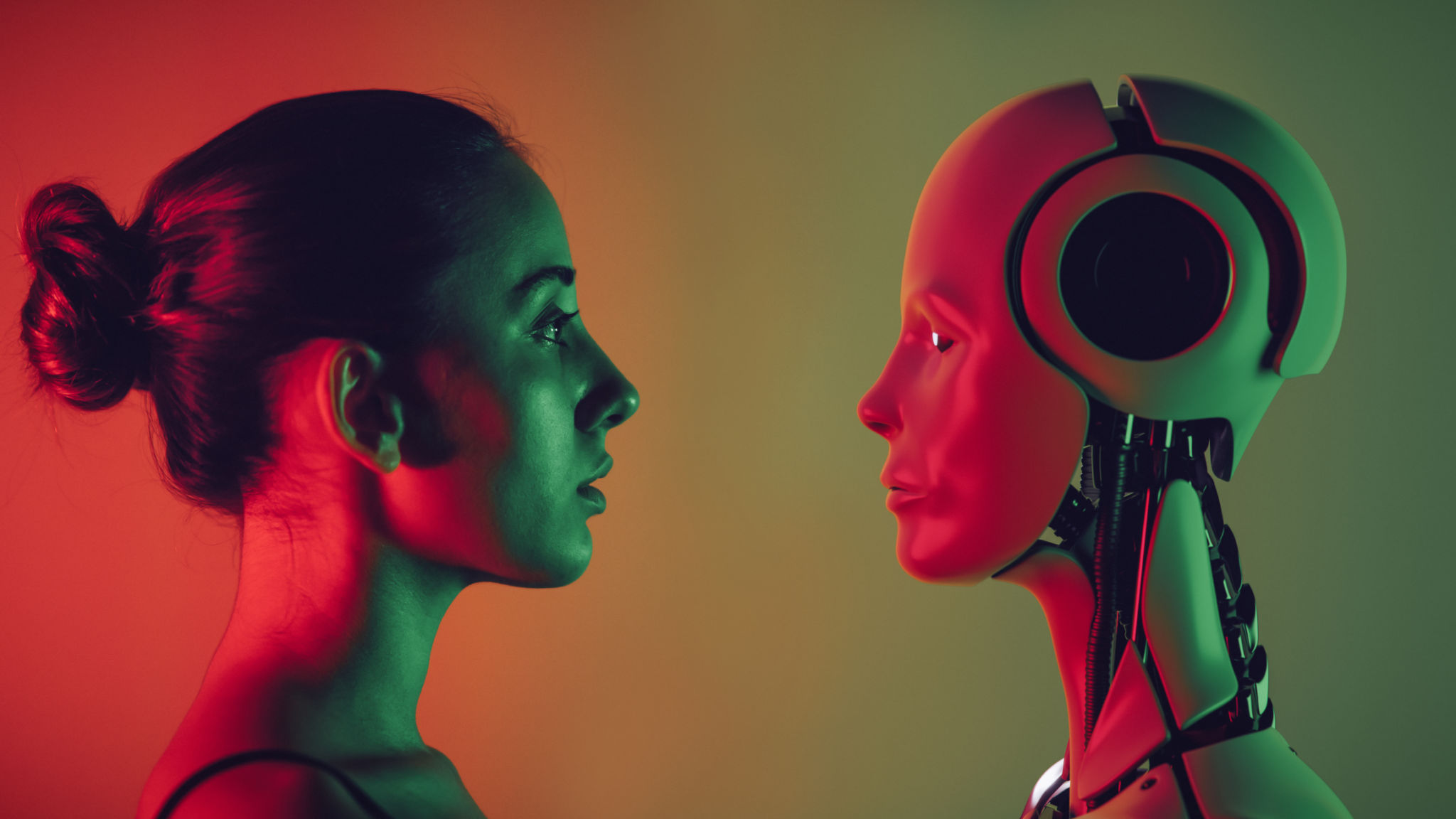
Integration with Existing Systems
Integrating AI solutions with existing systems presents a significant challenge. Legacy systems may not be compatible with new technologies, leading to integration hurdles that can delay AI deployment.
Solution: Adopt a Phased Approach
A phased approach to integration can help mitigate risks. Start by identifying pilot projects that offer quick wins and gradually scale up as the organization becomes more comfortable with AI technologies. Employing middleware solutions can also facilitate smoother integration between old and new systems.
Ethical and Bias Concerns
AI systems can inadvertently perpetuate biases present in training data, leading to unfair or unethical outcomes. Addressing these concerns is crucial for maintaining trust and ensuring the responsible use of AI technologies.
Solution: Implement Fairness Measures
Organizations should implement fairness measures by regularly auditing AI systems for bias and ensuring diverse representation in training datasets. Transparency in decision-making processes, along with engaging diverse teams in development, can help mitigate ethical concerns.
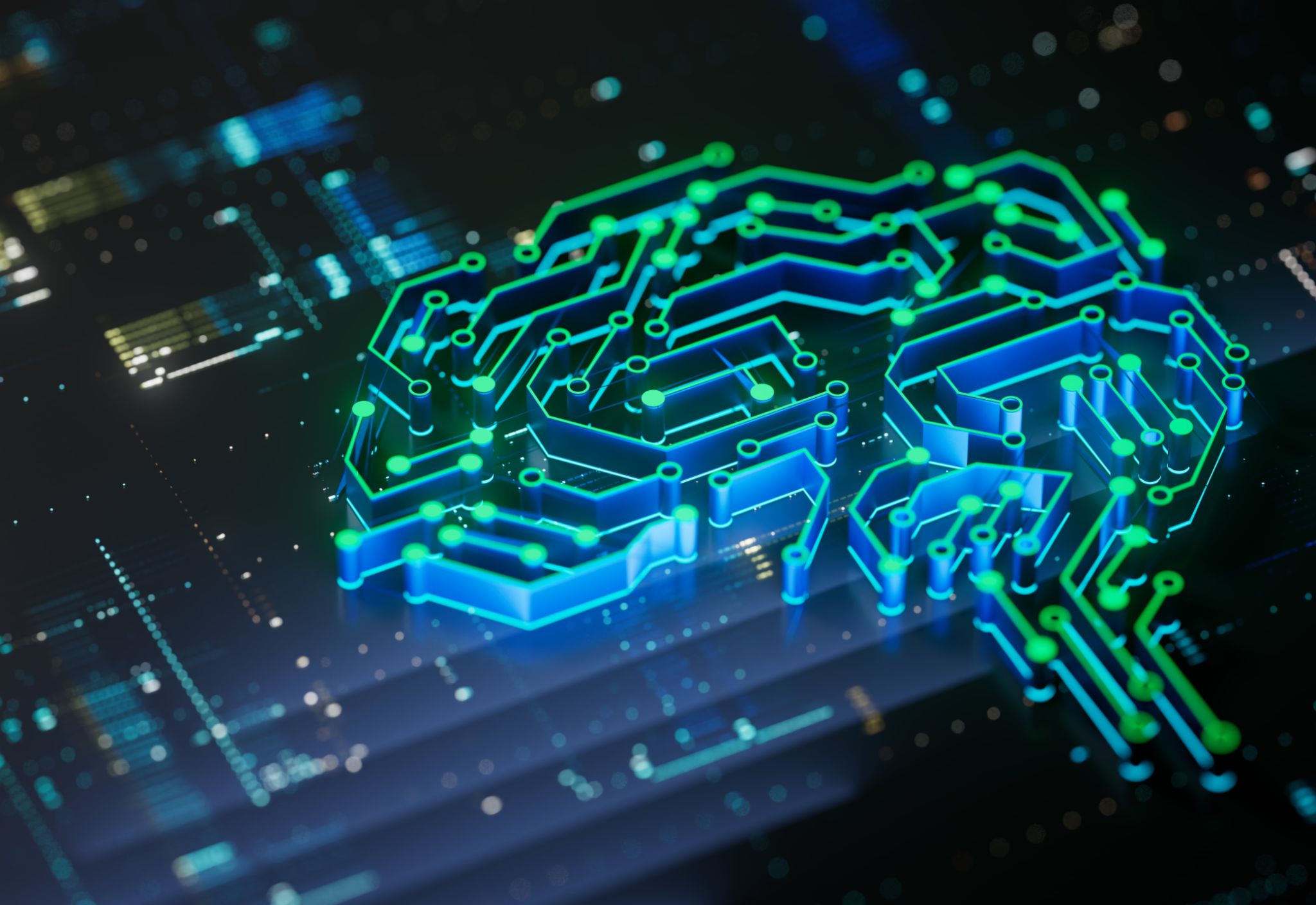
Conclusion
While AI implementation presents several challenges, they are not insurmountable. By focusing on data quality, addressing the talent gap, ensuring seamless integration, and maintaining ethical standards, organizations can effectively harness the power of AI. As technology continues to evolve, those who proactively address these challenges will be well-positioned to lead in the AI-driven future.